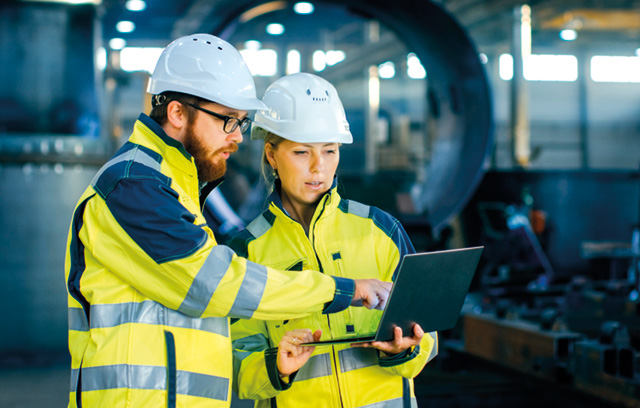
Marek Lukaszczyk, European & Middle East Marketing Manager at motors and drives manufacturer WEG, explains how businesses can get the most out of ‘Maintenance 4.0’ to improve equipment reliability.
Maintenance has always been challenging for industries such as chemical processing, oil and gas, mining, metals and pulp and paper. These asset intensive industries have been turning to new technologies in their efforts to increase the reliability and availability of their equipment, while keeping maintenance costs under control.
According to one industry report, digital transformation in the manufacturing market was valued at $263.93 billion in 2020 and is expected to reach $767.82 billion by 2026. However the deployment of technology to optimise maintenance has been a significant challenge.
Manufacturers that are ahead of the digital adoption curve have focused heavily on investing in plant connectivity and successful maintenance regimes to safeguard critical assets. In fact, it is often argued that, to minimise downtime effectively, maintenance technologies should be the most important aspect of digitalisation.
‘Maintenance 4.0’ is the phrase used to describe the digitalisation of maintenance and includes a holistic view of sources of data, analysis and recommended actions to ensure asset function and management are digitally assisted. Crucially, these technologies must be able to diagnose problems faster than a human engineer, often using artificial intelligence and machine learning techniques to do so.
High uptime is critical to ensure a return on investment for equipment in industries that often involve unpredictable circumstances, hazardous conditions and machine stress. While manufacturing companies have been adopting predictive maintenance approaches for decades, how can they get the most out of their programmes and move towards predictive maintenance maturity?
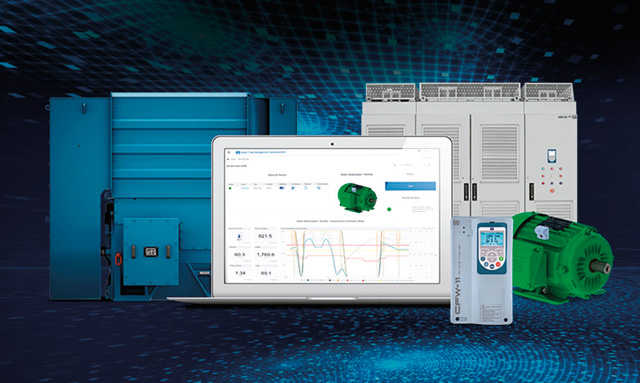
Insufficient data is often cited as a barrier to rolling out large scale predictive maintenance, along with prioritisation issues in terms of selecting which assets to include. The sheer volume of motors, drives, gearboxes and other assets in industrial facilities makes maintenance a hugely complex operation.
However, technological developments such as more affordable sensors, higher data availability, increased processing power and a stronger pool of technical partners with innovative digital platforms have made predictive maintenance more accessible.
Often, the problems that cause unplanned downtime in industry are hidden and can only be identified by monitoring vibration, heat and other outputs of industrial assets. For instance, in motors, common causes of failure include bearing wear, shaft misalignment and winding insulation, all of which can be identified through vibration and temperature analysis.
WEG’s own Motion Fleet Management (MFM) digital tool was developed for this reason. MFM can collect data from a range of different assets. Using cloud computing, operators are able to access the operational status of equipment including motors and drives in any industry or location. MFM collects and processes data regularly both at the edge and in the cloud to generate important information on asset operation.
Operators can use these insights to develop predictive maintenance plans and carry out condition-based maintenance. Periodic data collection gives a more thorough overview of asset condition, removing the need for maintenance teams to carry out observations and collect data manually.
Having access to this data more frequently can allow maintenance teams to make informed decisions quickly and minimise unplanned stops. This is especially important in asset intensive industries, where failure can result in loss of production.
Overcoming the challenges of predictive maintenance begins with a clear understanding of a manufacturer’s asset base and its reliability goals. Moving towards high levels of predictive maintenance maturity requires careful planning and the right advanced digital tools.