Condition monitoring is finally a reality for manufacturers of all shapes, sizes and sectors thanks to advances with Industry 4.0, automation and AI technology, says Senseye chief executive and founder Simon Kampa
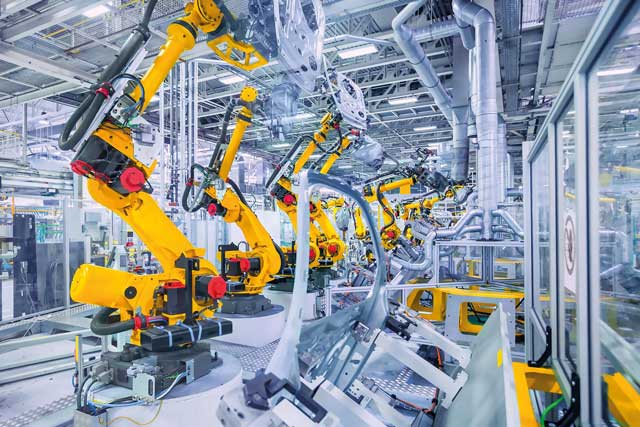
The roots of today’s data-driven approaches to condition monitoring first emerged in the aerospace and defence sectors over 30 years ago. It was an exhausting, manual exercise in pattern recognition, which relied on experts in data analysis to take readings from the machines, review the information they gathered and spot the crucial signs that a known fault might occur again in the future.
Industry 4.0 and cloud computing allow condition monitoring to take place automatically on a much greater scale. Software analyses vast quantities of data taken from existing machine sensors to automatically diagnose failures and provide the remaining useful life of machinery.
Much like the gathering of machine data, this analysis would have once been a highly manual endeavour, involving teams of data scientists. More recently, however, organisations such as Senseye have developed intelligent software to automate this activity.
The scalability this provides allows coverage of an organisation’s assets to be expanded, while reducing the risk for the monitored equipment. It is now possible to monitor much more than just the most critical assets.
Currently, the number of assets one person can monitor is likely to vary from between 50-100 a day. With increasing automation, the number of assets monitored can grow into the thousands. By automating 90% of the analytical tasks that the diagnostic system engineer performs, companies are able to address changes in the levels of critical assets in their organisation, without a tenfold increase in their labour costs.
A key factor in scalability is to make sure that the solution is sufficiently generic that it can be applied to different types of machines. Rather than creating bespoke algorithms for each machine type, start with a series of generic algorithms that will then be fine-tuned by the AI.
Progress continues to be made in refining and improving the power of the algorithms. Senseye’s AI has been refined to spot the earliest signs of machine failure – up to six months in advance.
This means that, for the first time, manufacturers can accurately predict (to within 24 hours given enough data) when a machine will fail and, as a result of this information, limit downtime even further, scheduling maintenance as and when it is needed.
The introduction of Senseye’s new Automatic Trend Recognition algorithms is just one example of this progress – able to monitor very gradual changes in the condition of industrial machinery over long periods of time. The algorithms analyse basic diagnostics data from machine sensors to spot small but significant variations in vibration, pressure, temperature, torque, electrical current and other sources that indicate deterioration in machine health.
Developed by Senseye’s own team of data scientists, the algorithms were designed so that they can be applied to any machine from any manufacturer, looking at the data that is already being produced. AI then fine-tunes the performance of each algorithm to maximise its accuracy.
The capabilities of condition monitoring have been greatly broadened by automating data collection and analysis, and providing usable information to engineers on the shop floor. The introduction of early-warning algorithms has revolutionised even the definition of predictive maintenance. Manufacturers can now monitor their machinery and plan its maintenance months in advance, helping to improve efficiency endlessly.